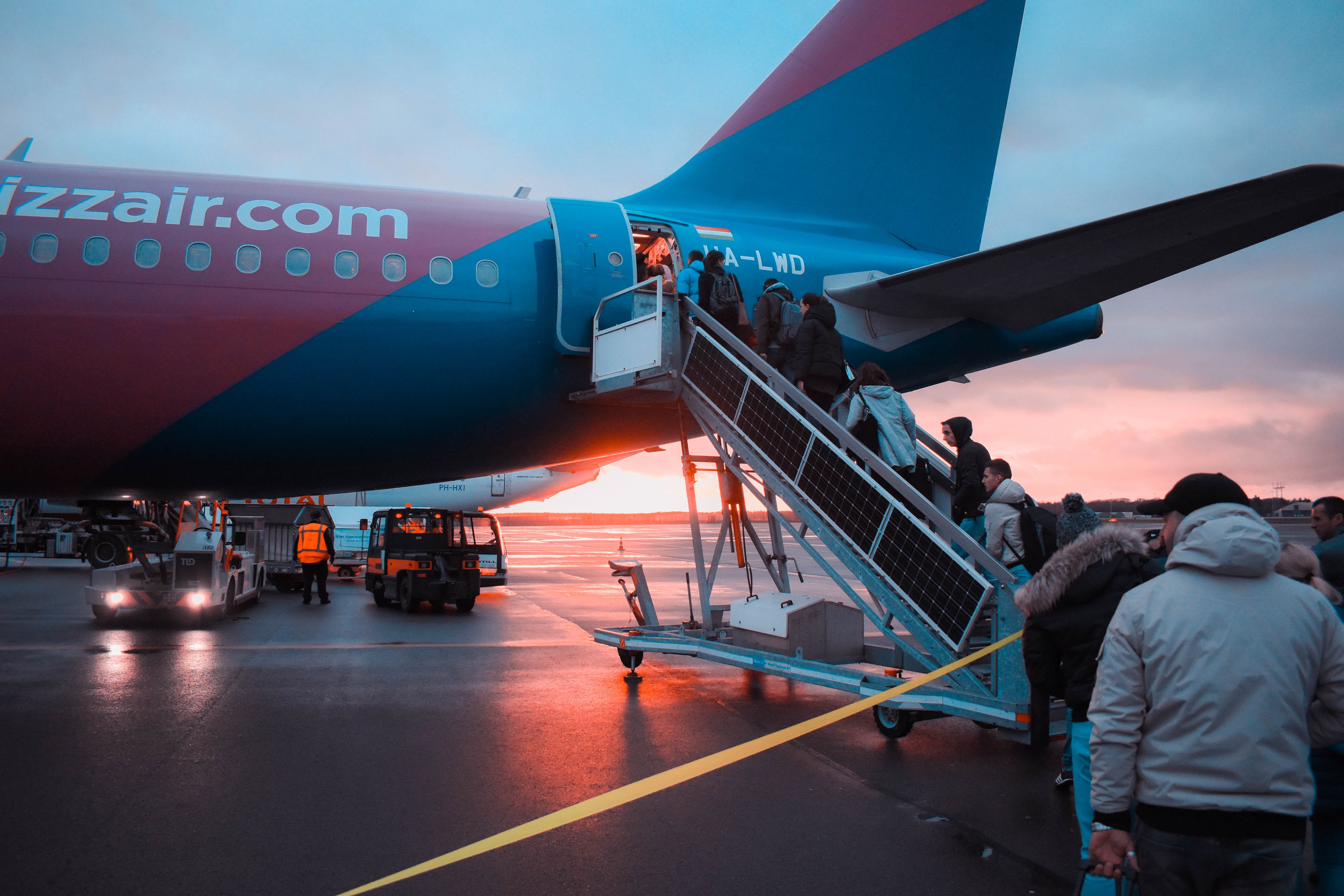
5 Ways Machine Learning Helps Travel & Hospitality Soar
85% of consumers say being treated like a person, not a number, is important to them. This is especially true in the travel and hospitality sectors, where the very process of traveling can be exhausting. Fortunately, these industries are working to personalize and improve the traveling and lodging experience through the use of AI. In this blog, we’ll explore the 4 ways travel is utilizing AI (including the orchestration of data) to improve customer experiences (CX) and increase conversions.
1. Improved Customer Experience & Service
Tourism has been on the rise globally, with the industry accounting for more than 1/10th of the world’s GDP and contributing $2.3 trillion to the U.S. economy in 2016. Additionally, digital innovation, in general, is what has propelled travel sales to grow from $500 billion in 2015 to an expected $800+ billion by 2020.
That said, digital innovation doesn’t mean robots showing you to your room or drones bringing you a poolside margarita (yet). No, it means using audience data to provide seamless customer experiences and easy user experiences. One way in which this has grown in the travel and hospitality industries is the use of machine learning chatbots.
These chatbots, which use Natural Language Processing (NLP), make it easy for customers to deal with the myriad of logistical issues that travel requires. For instance, a traveler-to-be may ask a chatbot in WhatsApp or Facebook Messenger, “Show me flight options from Los Angeles to New York for Christmas.” Then adding, “Use frequent flier miles for the purchase.” And if the chatbot has access to enough customer data, it can return a highly customized list of recommendations, greatly increasing the likelihood of a purchase.
Kayak is currently the gold standard to follow for marketers hoping to improve how customers search and purchase with chatbots. Through multiple channels, Kayak takes simple requests — “I want to fly to Mexico City in September” — and will help travelers book flights, hotels, cars, or just find someplace to explore. And the conversation does not stop at the point of sale. They also send travel plan updates to customers via messenger.
Meanwhile, older companies like Amtrak have embraced chatbots as well. Amtrak has a chatbot named Julie that uses NLP to help customers easily book tickets. Just type what you need— “I need a ticket to New York”— and “she” will ask you where you’re departing from, if it’s one-way or roundtrip, and automatically show you a page with the relevant fare choices. It’s a lot easier than using the pull-down menu interface and trying to remember train station codes.
2. Ameliorating Problems
We’ve all been there. An issue has come up in our travel plans, and we need answers! There is very little in this world less frustrating than being put on endless holds, yelling at a robot on the phone to speak to an operator, and being passed around from person to person in a never-ending loop of unhelpfulness. Fortunately, many travel companies are aware of this issue and are working hard toward addressing customer difficulties through machine learning.
Dutch airline KLM, for instance, is using machine learning to field customer service requests via social media, often without the intervention of a live agent. KLM says its team of 250 social media service agents has about 30,000 conversations a week. Customers contact KLM through Twitter, Facebook, and WhatsApp and a machine learning platform analyzes customers’ questions. Then, it instantaneously returns suggested responses that show up on the screen of a customer service agent. The agent could accept the answer, reject it, or send a personalized response. Because their customer service process uses machine learning, it’s gotten more accurate over time, allowing KLM to automate the most common questions and save the most thorny stuff for human agents.
3. Better Recommendations
In a survey of 2,000 United States travelers, Mindtree found that nearly 9 in 10 respondents are more loyal to a travel provider that offers relevant deals personalized to them. With advances in machine learning and custom-built algorithms, travel companies must invest in recommendation engines to increase opportunities for cross-selling. By collecting information from travelers’ recent searches, companies can create a recommendation algorithm to offer suggestions for add-ons to their next trip or emails with discounted flights to destinations they prefer.
United Airlines has been particularly successful in using machine learning to personalize its marketing materials. The airline tracks customer behavioral data and pairs it with historical data such as search destinations and purchases to create detailed customer segments. Then, the airline adjusts specific landing pages, layouts, and content on its websites to increase sales.
Another great example is Expedia. After analyzing thousands of data points, Expedia discovered that people search about 48 times across travel sites before booking a flight. Expedia’s new flight recommendation engine simplifies this process by analyzing customer booking patterns to provide more relevant searches with a list of alternative routes and the ability to compare query results with past searches.
4. Advanced UX Personalization
With the right orchestration technology, data can impact travel recommendations and create dynamic UX personalization as well. According to Salesforce, 75% of consumers agree they expect companies to provide a consistent experience wherever they engage, be it social media, mobile, on-site, or in-store.
Take for example United Airlines, which uses customer actions and historical data (like previous purchases and travel destinations) to make changes to its website, landing pages, web copy, and on-screen layouts to reach specific consumer segments.
United does the above by assessing more than 150 variables about a customer, in real-time, to deliver dynamic personalization of their website in about 200 milliseconds based on the individual. According to United’s vice president of e-commerce and merchandising, Scott Wilson, this data-driven, customer-centric strategy has led to a 15% increase year-over-year in ancillary revenue.
5. Price Optimization & New Revenue Streams
Predictive analytics, a subset of machine learning, is another huge asset for the travel industry. Using a combination of large data sets and custom-built algorithms, travel brands can create dynamic pricing for reservations based on customer booking patterns, the weather, average daily rates, and more.
Southwest Airlines, for example, uses aggregated customer data to promote its services, products and featured offers across channels and devices to reach the right traveler. Dan Landson, a company spokesperson, explains how the airline leveraged customer behavior data to increase revenue, “We look at the city pairs that are being searched to help us determine what type of service we should offer on a specific route.” In doing so, Southwest’s customer and loyalty segments have grown year-over-year, with Landson attributing that growth to intelligent, data-based targeting.
Prior to its acquisition by Marriott in 2016, Starwood Hotels & Resorts Worldwide spent $50 million on developing its demand forecasting system. This system used AI to learn how to price rooms more efficiently to boost revenue and profits. This was a marked improvement over the old method of room pricing, which might be updated 2-3 times a day. Starwood’s software allowed it to update room pricing minute to minute, based on a dizzying number of calculations behind the scenes in Starwood's data center. It cross-checked past and present reservation data, booking patterns, cancellation rates, occupancy data, room type, and daily rates, as well as whether you're a solo traveler or a group. The system also analyzed external data, such as competitive prices, weather, and other sites’ booking patterns. And it worked. Building an algorithm capable of processing these factors led to a 20 percent improvement in demand forecasting and led to Starwood’s ultimate Marriott acquisition.
A Shining Example in Airbnb
From stellar customer service to personalization, Airbnb leverages data to continuously strengthen its relationship with guests and hosts. According to Airbnb, consumer preferences are broken down into four categories:
- The Behavioral Aspect: How an Airbnb user interacts with their site online
- Dimensional Factor: The device used, language, and location preference
- Sentiment: Their reviews, survey results, and ratings
- Imputed: Sorts location preference of traveler, i.e. city vs. local town
By layering the above types of data, Airbnb can improve the types of travel recommendations it provides when customers search their platform, including factors like proximity to attractions, types of amenities, and more.
Airbnb uses its 11 petabytes of data to help hosts as well. Through machine learning, Airbnb lets hosts know which calendar dates are likely to be booked at their current price as well as which dates may not be booked, so they can receive more dynamic pricing suggestions. This, in turn, helps hosts understand when to make changes to their pricing around big events (think, SXSW in Austin or New Year’s Eve in NYC) and can also help them earn more money in the long run.
Airbnb also conducts “experiments,” or, A/B tests, on their product to ensure constant improvement. That said, it can be difficult to tell the exact impact of a particular product change since, as Neil Patel explains, there are several aspects of how Airbnb works that “make it more involved than simply changing the color of a button and measuring what happens.” Patel continues,
Users can browse Airbnb whether they’re logged in or not. This can make it a challenge to tie actions to a particular user. It’s also possible for them to browse on their mobile device, then come home and complete the booking process on their home computer. Furthermore, a successful booking may depend on the guest’s request and how responsive the host is–things that are beyond Airbnb’s control.
Data isn’t a magic band-aid that will solve all your brand’s problems. Even Airbnb struggles with optimizing for things like cross-channel browsing or host responsiveness. But, using data to provide stand-out customer experiences can get easier as long as you have the right tools to analyze it holistically.
How Lineate Can Help
According to McKinsey, travel companies have a 23x higher chance of customer acquisition if their strategy is data-driven. Lineate’s data orchestration solutions help travel brands like Classic Journeys centralize disparate data sources to gain 360 audience insight and launch highly-targeted campaigns across every channel. The result? An ability to understand who your customers are, where they come from, and how to reach them at the right time and place. To see how data orchestration and machine learning can take your marketing strategy to new heights, book a free consultation with our SA team today.
Share:
Got a project?
Harness the power of your data with the help of our tailored data-centric expertise.
Contact us